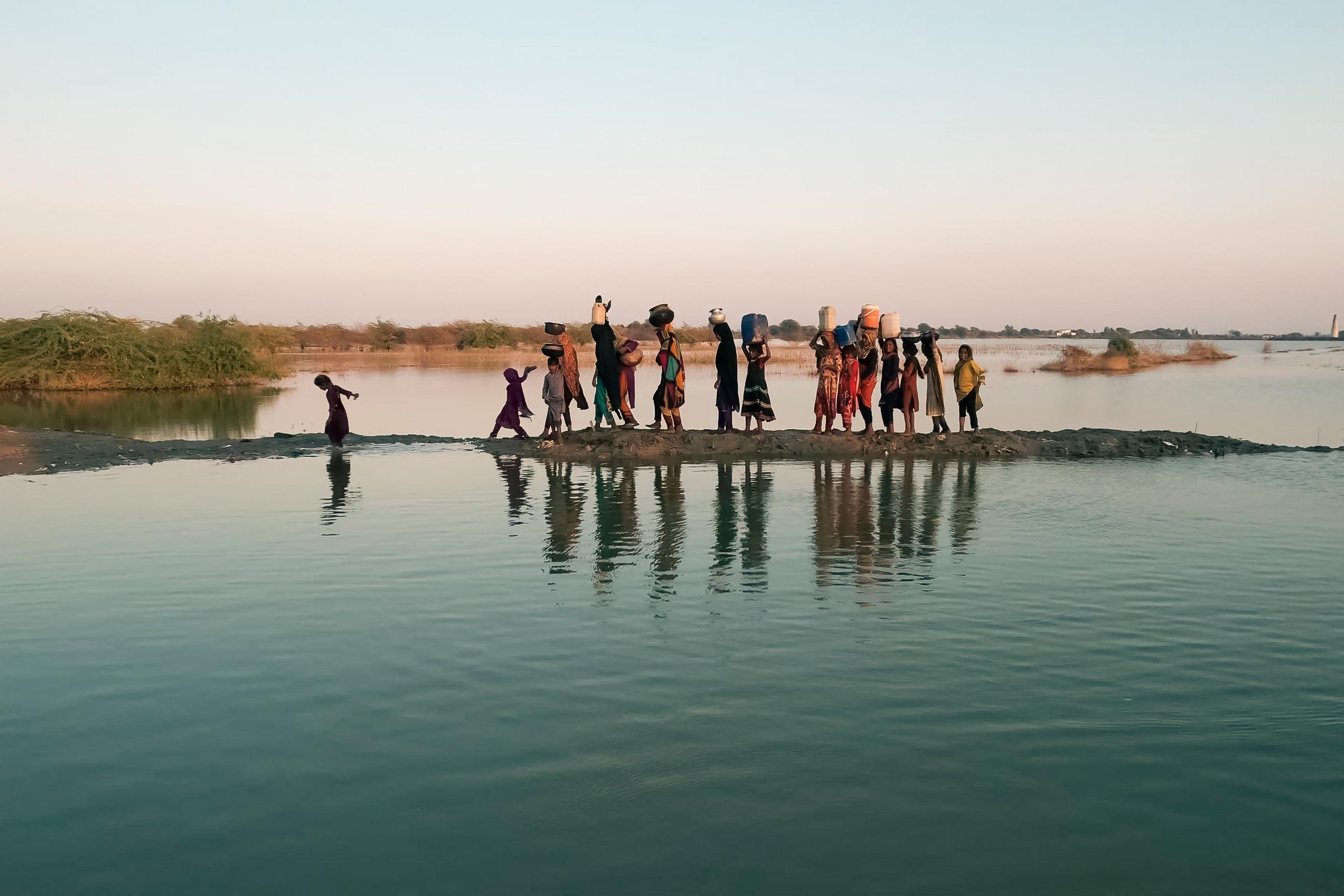
Expert analysis
06 August 2024
How AI is advancing socioeconomic insights into disaster displacement
We cannot afford to ignore an alarming trend: the number of disaster-induced displacements is on the rise, hitting hardest in regions already grappling with economic and social challenges.
Faced with destructive floods, storms and landslides, millions are forced to abandon their homes and means of survival. To better understand and anticipate disaster displacement, our investigative journey employs a type of artificial intelligence (AI) with transformative potential: machine learning. This innovative approach utilizes advanced statistical techniques to forecast and, over time, mitigate the disruptions caused by extreme weather events in a changing climate. It leverages the growing abundance of available data for this purpose.
What really triggers forced displacements?
When we think about climate change, we often visualize melting ice caps and desiccated fields. However, there's an underrepresented narrative that is just as compelling—one of human populations who are forcibly displaced due to relentlessly intensifying extreme weather conditions.
At first, it seems like "natural" disasters are clearly the cause, but the reality is more complicated. The extent of displacement is not just determined by the severity of disasters, but also by the vulnerability of the people affected by disasters. With artificial intelligence and other forms of machine learning, we now have new tools to help us understand the realities of how climate, weather and displacement overlap.
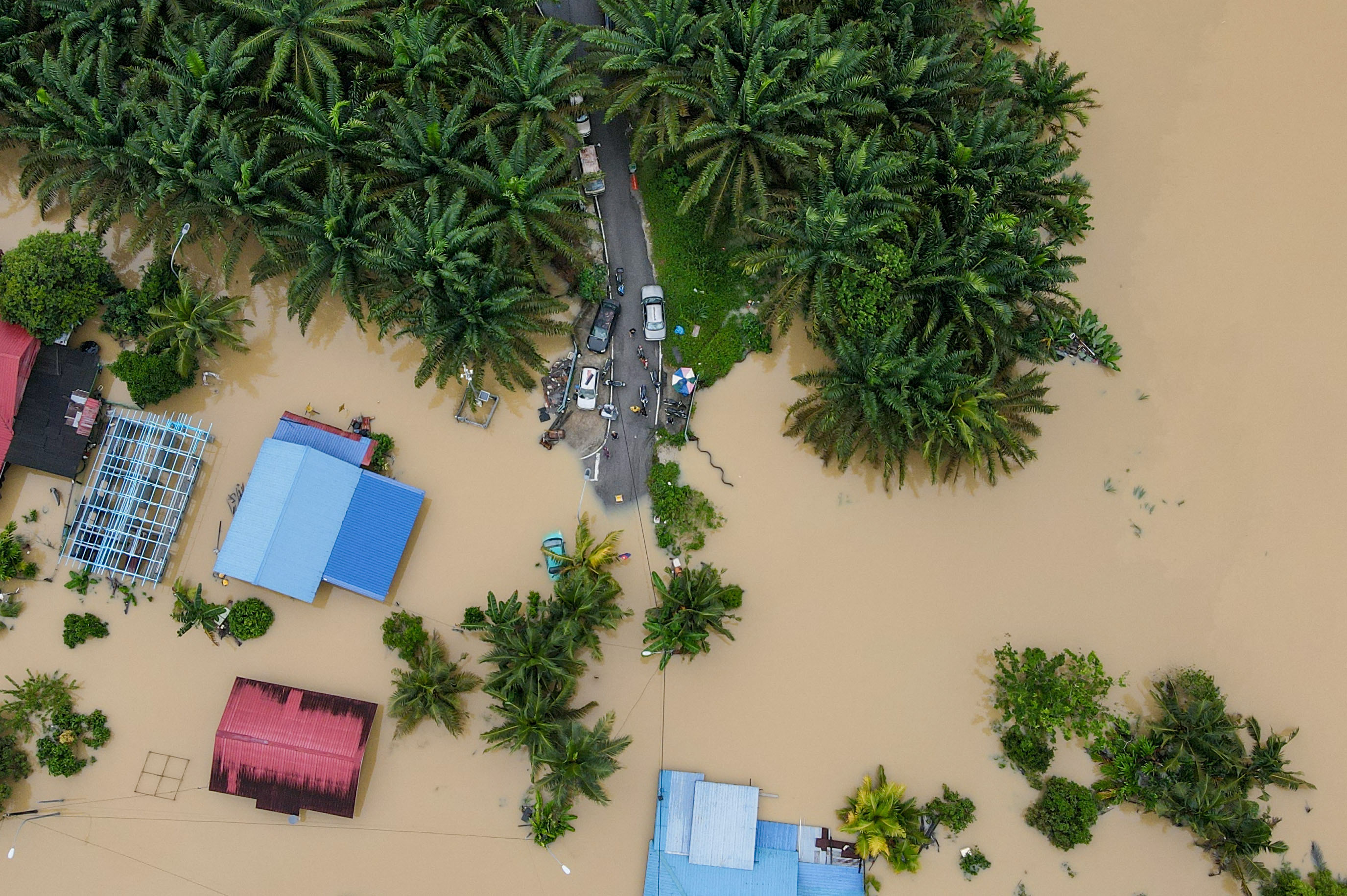
The analysis of our data presents a complex picture of vulnerability and urgency: regions suffering from poverty, often on the front lines of extreme weather events, bear a disproportionate rate of displacement. This underscores the inequitable impact of climate change. When exposed to similar hazard intensities, like equivalent rainfall during disasters, the number of movements of people is heavily influenced by their income levels.
Regions with higher incomes witness notably fewer displacements, indicating an unequal burden borne by economically vulnerable communities. This confirms that income, and by implication the resources available for disaster preparedness and recovery, play a decisive role in determining the impact of weather related-related disasters.
Communities with strong infrastructure and economic resources often bounce back swiftly from disasters, while those in economically disadvantaged areas likely face more prolonged and disruptive displacement. Education, however, is as a catalyst for change. By cultivating knowledge and skills, education fortifies the capacity of individuals and entire communities to withstand and adapt to the relentless challenges posed by living in a changing climate.
How can artificial intelligence predict displacement?
Forecasting displacements is an intricate task, shaped by a multitude of intertwined factors. Machine learning serves as a powerful tool to decode these complexities, extracting nuanced patterns from extensive sets of observational data.
Our machine learning approach relies heavily on the abundance and quality of data to achieve optimal performance. Its capacity to handle varied inputs—from climatic conditions to socioeconomic indicators such as income and educational expenditure—is crucial. These methods filter through the data deluge to highlight the most significant indicators.
Our approach extends beyond merely establishing correlations; we endeavor to construct a predictive framework enriched by environmental, societal, and economic variables. Central to our methodology is explainable AI. This vital aspect of machine learning unravels the logic behind our predictive models.
It doesn't just project outcomes—it illuminates the rationale behind each prediction, fostering transparency and trust. For policymakers and communities at the forefront of climate change, this level of insight is key for steering decisions that aim to anticipate and navigate the ramifications of displacement.
Random forests: What is it?
Random forest is a versatile and powerful machine learning algorithm that leverages the collective wisdom of multiple decision trees to make accurate predictions.
Leveraging the combined prowess of ensemble machine learning models, such as random forests and gradient boosting machines, we are enhancing our understanding of the complexities of displacement induced by weather-related events.
These advanced models enhance our predictive capabilities by combining multiple simpler models, similar to an interdisciplinary team of experts pooling their collective insights. Each statistic and data point represents a life disrupted, a community's resilience tested to the limit, and a future suspended in uncertainty.
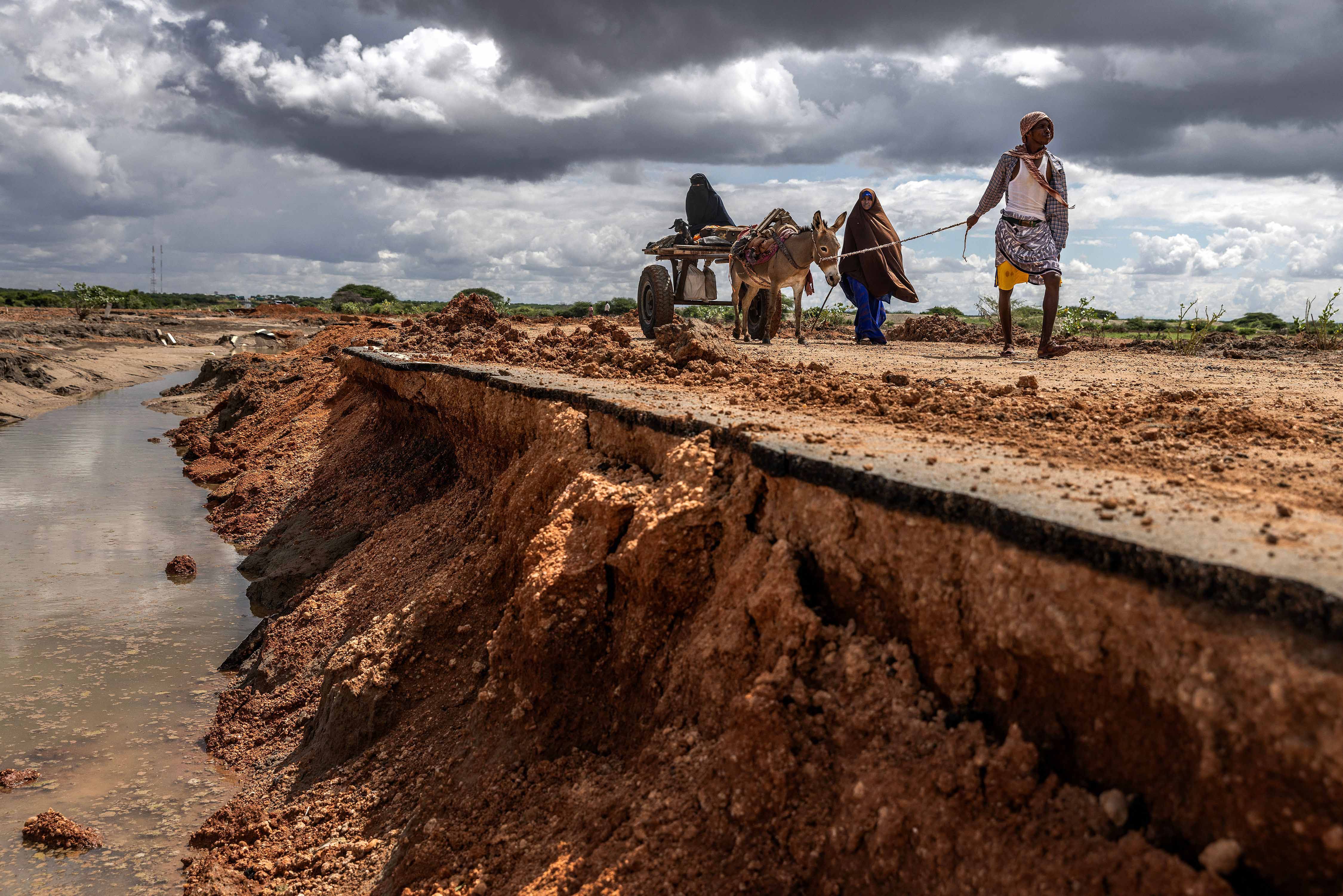
How can artificial intelligence help us prevent and respond to displacements?
Prediction offers a ray of hope in the face of the growing impacts of climate change and resulting disaster displacement. By harnessing machine learning and the insights unveiled by explainable AI, we can not only predict but also mitigate the impacts of weather-related hazards-induced displacement in a changing climate. This offers the potential for a more proactive approach to looming challenges.
Scatter plots of Shapley values versus precipitation (A) and area (B), and box plots of the treatment effects obtained with causal forests (C, D)
Amidst the complex interplay of factors driving displacement and equipped with growing amounts of displacement data, we are left with a crucial question: How will we harness this knowledge to stem the rising tide of displacement related to disasters caused by natural hazards and the adverse impacts of climate change? The answer lies in our collective resolve to transform understanding into meaningful, impactful action.
Our data-centric approach holds the promise of ensuring a more equitable distribution of resources and support in response to extreme weather events. Guided by our findings, we can formulate more potent policies, build more robust shelters, deliver aid faster and foster communities that are resilient and adaptable in the face of adversity.
What data is used for forecasting displacement?
IDMC’s Global Internal Displacement Database (GIDD) is a unique global repository providing verified, consolidated, and multi-sourced estimates of movements of people by disasters. Its database includes records for more than 400 million movements of people dating back to 2008, meticulously categorised by hazard type, date, and location.
Building on this comprehensive data, the collaboration between IDMC and the University of Valencia, under the umbrella of the DeepCube Horizon2020 European project (Explainable Artificial Intelligence pipelines for big Copernicus data), has been a successful partnership. Initiated in February 2020, we have focused on examining sudden-onset disaster events from 2016 to 2021, such as floods and storms, spanning a dataset of around 2,400 figures.
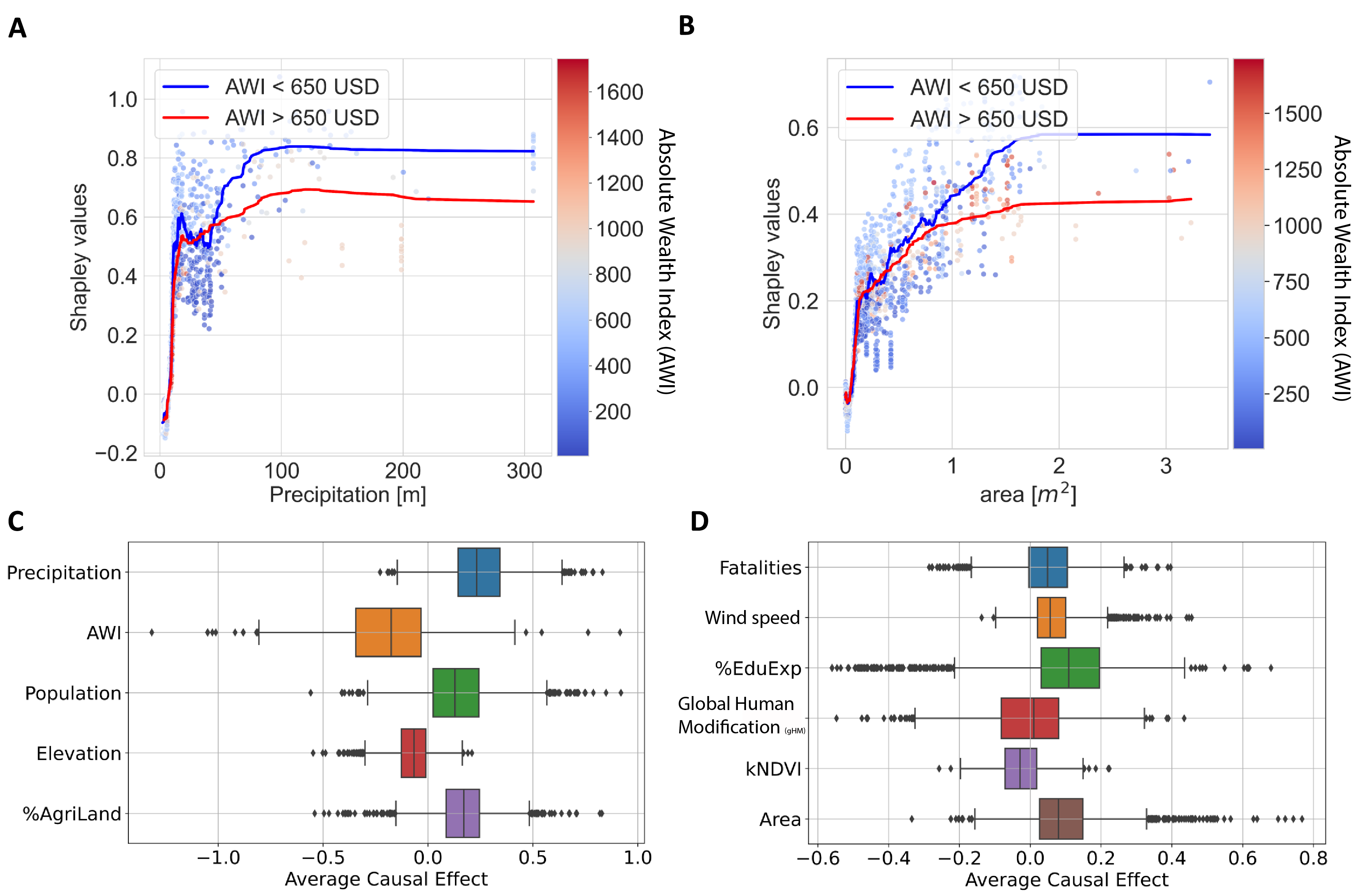
In the bottom graphs (C, D), we show the distribution (median and spread) of the causal relationship between the target (i.e., NDP) and each of the covariates considered one by one as treatments.
For more information read the full article “Exploring interactions between socioeconomic context and natural hazards on human population displacement” in Nature